How can teachers use learning analytics to improve instruction?
In our preceding blog post, we explored the realm of Teaching Analytics. Now, in the concluding part of this series, we shift our attention to Learning Analytics. This final blog systematically examines data derived from students and learning environments. The objective is to enhance learning outcomes across various educational levels through insightful analysis and application of the gathered information.
Learning Analytics
Learning analytics endeavors to scrutinize data from students and learning environments to bolster learning outcomes across diverse educational tiers. Despite being a relatively recent field, learning analytics has attained a notable level of maturity, particularly within the domain of higher education. However, the scope of research in learning analytics has been limited, with minimal attention directed towards educational levels beyond higher education, such as high schools. This paper presents the outcomes of a systematic literature review (SLR) on implementing learning analytics in high schools. The SLR methodically progressed through four stages: the search, selection of pertinent studies, critical evaluation, and extracting relevant fields, encompassing primary objectives, approaches, techniques, and challenges associated with integrating learning analytics in high school settings. The findings reveal that, within this context, learning analytics applications predominantly manifest as small-scale initiatives rather than widespread institutional adoption. Building upon these study outcomes and existing literature, this paper posits future avenues for research and development, seeking to broaden the scope and scale of learning analytics applications within high school environments.
Learning analytics, as defined, entails the measurement, collection, analysis, and reporting of data concerning learners, learning environments, and contexts, with the overarching goal of understanding and optimizing the learning process and its associated territories. Online learners leave valuable data traces through their activities, which learning analytics can systematically gather from various sources. Subsequently, this data is analyzed to generate meaningful insights and visualizations, catering to the informational needs of institutional managers, teachers, and learners.
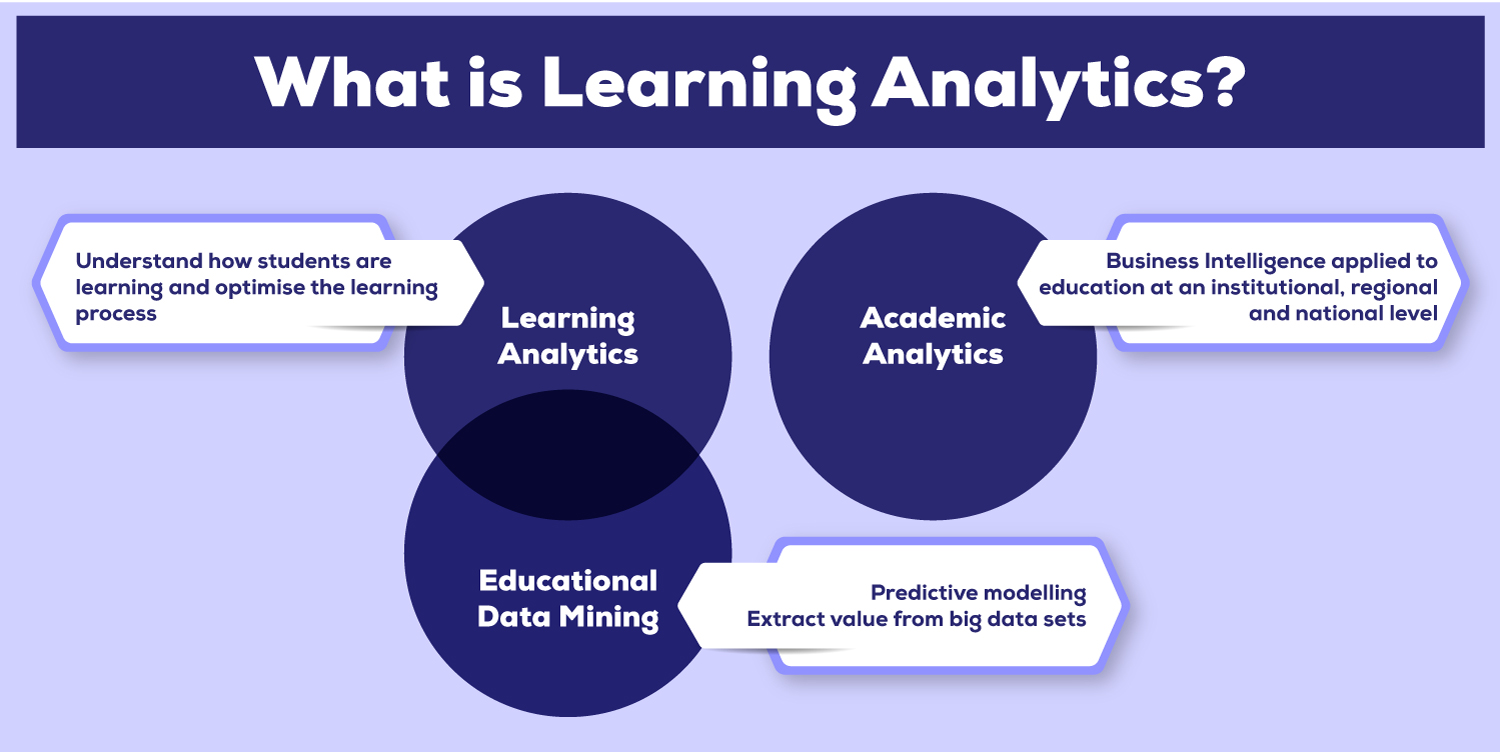
Learning Analytics And Technology
Technology has become indispensable in fostering more compelling educational experiences for students and instructors in recent years. The proliferation of online learning environments, including learning management systems, student diaries, library systems, digital repositories, and academic systems, has significantly increased the volume of data generated throughout the educational process. Capitalizing on the potential for analysis inherent in this data, learning analytics has emerged as a specialized field focusing on systematically collecting, analyzing, and reporting data about learners and the contextual parameters influencing the learning process. Applying learning analytics holds tangible benefits for students, teachers, and educational institutions. The extensive dataset encompassing student demographics, grades, and behavioural patterns affords opportunities for personalized retention strategies and academic success, moving away from standardized approaches to cater to the individualized needs of each student.
While learning analytics has been extensively researched and embraced within higher education institutions, the same level of adoption is notably absent in other educational contexts, especially high schools. This discrepancy is limiting, given the potential richness of information that can be gleaned from the data generated in these educational settings. The increased capabilities of educational data mining necessitate a closer examination of how learning analytic tools extend their influence beyond the classroom, shaping activities, curriculum, and pedagogy, as underscored by Brown’s call for investigation in this domain.
Numerous educational challenges within the high school context involve all stakeholders engaged in teaching and learning processes. Learning analytics emerges as a strategic tool to address these challenges, encompassing issues such as school dropout rates, impediments to effective collaboration among students, the cultivation of scientific argumentation and writing skills, and the development of computational thinking—an emerging competency pertinent to this age group. Through learning analytics, teachers can receive support in comprehending student practices, variations in classroom dynamics, and monitoring students’ motivation levels. Managers and decision-makers, in turn, can leverage learning analytics to identify students facing vulnerability in terms of timely graduation and in crafting curriculum aligned with students’ needs and expectations.
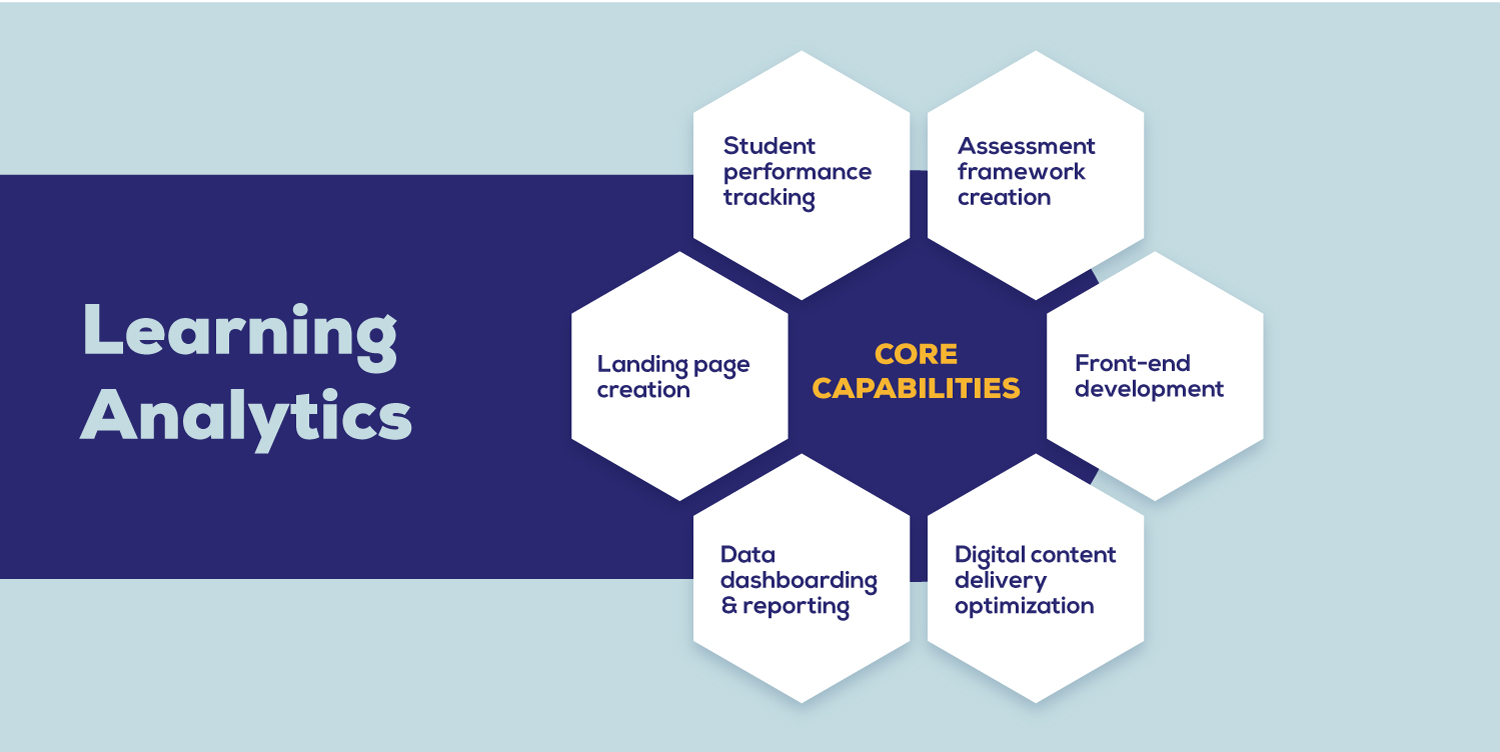
The Systematic Literature Review (SLR)
Considering the context above and the existing literature reviews emphasizing the potential of learning analytics in various educational settings—higher education, professional and workplace learning, vocational education, and massive open online courses—an evident gap exists concerning its application in the high school context. Against this backdrop, this paper presents a systematic literature review (SLR) that delves explicitly into the applications of learning analytics in high schools. The SLR facilitates the identification, evaluation, and interpretation of prior works, elucidating methods, tools, and the utilization of learning analytics in this unique educational setting. More precisely, this review offers a comprehensive overview of primary approaches, educational objectives, techniques, and challenges associated with the intersection of learning analytics and high schools.
Despite the evident advantages inherent in the application of learning analytics, there needs to be more literature exploring its benefits within the high school context. While learning analytics has the potential to address numerous challenges high schools face, including student dropout rates and the cultivation of computational thinking abilities, its consistent utilization across institutions still needs improvement. This limitation may stem from the dearth of studies scrutinizing the contextual relevance and potential of learning analytics in high schools, coupled with a lack of engagement with diverse stakeholders in the adoption process— a practice prevalent in higher education. Therefore, there exists a need to bridge the gap between technological capacity and tangible improvements in teaching-learning experiences. In this context, it is imperative to identify and analyze reports of incidents that elucidate the practical consequences of applying learning analytics in high school settings.
Several literature reviews on learning analytics have been published over the past 11 years since the inception of the LAK conference. These reviews have synthesized methods and techniques for data analysis through learning analytics based on papers published between 2010 and 2018. Furthermore, some studies have conducted bibliometric investigations of the field, analyzing publication counts, citation counts, co-authorship patterns, citation networks, and term co-occurrence. Common themes in these reviews highlight higher education institutions as frequent keywords.
Besides these general reviews, previous systematic literature reviews (SLRs) have focused on specific methods, particularly those related to developing visualizations and dashboards. These studies present current applications and tools for crafting learning analytic visualizations, elucidating how students and instructors can benefit from learning analytical dashboards in practical settings and delineating the associated challenges and future research directions.
Beyond merely predicting final grades, the application of learning analytics in high school settings extends to forecasting school dropout rates, identifying precursors to potential middle school failure in its nascent stages, and providing valuable insights to educational departments and policymakers for anticipating both the graduation and dropout student populations.
Furthermore, a second prevalent educational objective involves the nuanced analysis of students’ learning processes. A substantial body of literature aligning with this objective focuses on scrutinizing students’ engagement in assessments and educational games by examining log data. Noteworthy examples include a comprehensive study conducted at a senior high school in northern Taiwan, where diverse data sources, including self-report models and activity logs, were gathered from 25 classes. The objective was to apply supervised and unsupervised lag sequential analysis (LSA) to examine students’ learning processes meticulously. Employed log data to assess students’ participation in computational thinking activities and the Go-Lab portal, respectively. The primary aim of these studies was to support students in reflecting on personal knowledge building by visualizing their log data. Another innovative application involved analyzing the behaviour of solo and collaborative student groups engaged with educational games. The evaluation focused on discerning differences in interactions between these two profiles based on in-game log data, representing a pioneering approach scalable to large student cohorts.
Moreover, the literature retrieved underscored the relevance of supporting teachers’ decision-making processes and reflective practices. One notable contribution in this realm proposed an approach elucidating how teachers’ behaviour influences classroom teaching performance. Various papers advocated for tools such as data visualization, real-time learning analytics, and computer-based assessment data visualization to empower teachers in making informed decisions. Visualization emerges as a central theme within learning analytics research and practice, categorized as distilling data for human judgment. Several instances showcased the practical application of visualizations to assist diverse stakeholders in high school contexts. The educator introduced the Visual Learning Analytics (VLA) approach, amalgamating learning analytics and visual analytics perspectives to comprehend educational processes. Applied to support a video-based teacher professional development program, the study compared the efficacy of conventional knowledge-based workshops with VLA-supported hands-on workshops. Results indicated that VLA-supported professional development effectively enhanced teachers’ methodologies and promoted dialogic teaching in the classroom.
The methodologies delineated in this section exhibit a substantial nexus with educational objectives, particularly those associated with predicting and enhancing students’ learning outcomes. The papers within this domain commonly employed conventional machine learning algorithms, including decision trees, naïve Bayes, support vector machines, logistic regression, and neural networks.
The prevalent utilization of decision tree algorithms and clustering techniques is noteworthy for investigating students’ learning processes. Traditional decision tree algorithms, specifically decision trees and random forests, featured prominently in seven and three papers, respectively. Only two pieces ventured into state-of-the-art decision tree algorithms: Adaboost and XGBoost. Furthermore, the k-means algorithm emerged as the predominant choice, employed in 75% of papers dedicated to clustering analysis.
As anticipated, incorporating natural language processing (NLP) techniques was discernible in papers centred on supporting writing activities. For instance, Allen et al. leveraged diverse language models and resources rooted in the start system to scrutinize discourse dynamics within a reading strategy. Researchers advocated using the MI Write system to evaluate written activities across diverse contexts.